Metallurgical Abstracts on Light Metals and Alloys vol.56
Exploration of Superconductivity in Three-elements Light Metal System
~Proposing Exploration Strategy and Assuming Superconducting Components by Machine Learning~
Narimichi Mokutani1, Yongpeng Tang1, Masaki Mito1, Kaname Matsumoto1, Mitsuhiro Murayama2 and Zenji Horita1
1 Graduate School of Engineering, Kyushu Institute of Technology, Kitakyushu 804-8550, Japan
2 Institute for Materials Chemistry and Engineering, Kyushu University, Kasuga 816-8580, Japan
[Published in Materia Japan Vol. 61 (2022), 870–877]
https://www.jstage.jst.go.jp/article/materia/61/12/61_870/_article/-char/en
E-mail: mitoh[at]mns.kyutech.ac.jp
Key Words: superconductivity, light metals, machine learning, high-pressure torsion, quasi-stable states
We explored new superconductors in the Al-Mg-Ti ternary system, along with the prediction using machine learning. High-pressure torsion (HPT) is used to produce the superconducting states, which is intrinsically quasi-stable. We found the superconducting states with the superconducting transition temperatures (Tc) of 7.3 K for a composition of Al:Ti = 1:2. The magnetic anomalies appeared more sharply when the sample was processed by HPT at 573 K than at room temperature. Magnetic anomaly was also observed at approximately 90 K, being supported by the prediction using the machine learning for the Al-Ti-O system. We conclude that Al-Ti oxides play an important role in the advent of the anomaly. Al and Ti are superconducting materials but their Tc values are quite low as 1.20 and 0.39 K, respectively. The creation of quasi-stable superconducting states using HPT would stimulate the study of superconductivity.
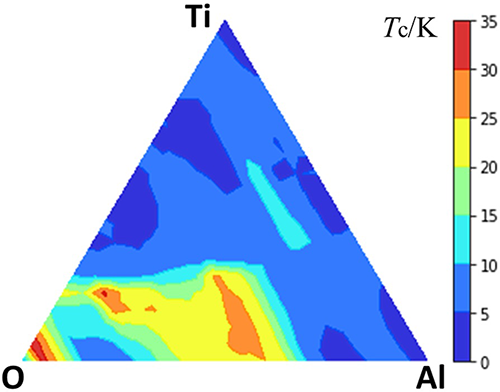
Mapping of Tc in an Al-Ti-O triangle, predicted by machine learning based on about 14 000 superconductor dataset, including cuprate and iron based superconductors.