Metallurgical Abstracts on Light Metals and Alloys vol.56
Fractographic Classification by Transfer Learning Considering Material Types
Tokuteru Uesugi*, Mitsuaki Kondou**, Naoyuki Hamada***, Shunsuke Kita*** and Tomotake Hirata***
*Graduate School of Informatics, Osaka Metropolitan University
**School of Knowledge and Information Systems, Osaka Prefecture University
***Osaka Research Institute of Industrial Science and Technolog
[Published in Journal of the Society of Materials Science, Japan, Vol. 72 (2023), pp. 376-383]
https://www.jstage.jst.go.jp/article/jsms/72/5/72_376/_article/-char/en
E-mail: uesugi[at]omu.ac.jp
Key Words: fractography, deep learning, transfer learning, convolutional neural networks, image classification
Fractographic analysis of fracture surfaces helps to improve the performance of mechanical pieces. In order to determine the causes that generated the fracture, it is necessary to classify a fracture into a failure mode. Experts in fractographic classification use visual evidence of textures and surface marks to determine the failure classification. The fractographic images for this task are obtained using a Scanning Election Microscope (SEM). With new advances in machine learning and artificial intelligence, specifically with deep learning and Convolution Neural Networks (CNN) becoming accessible, it is becoming possible to automate fractographic classification. This study aimed to improve the accuracy of fractographic classification using CNN by considering the material types. We proposed a Stepwise Selection of Source Classes (SSSC) to perform transfer learning considering the material types. The proposed method was applied to classifying fractographic SEM images into seven groups of fracture surfaces in alloy steels, austenitic stainless steels, and copper and copper alloys. The existing method, which does not distinguish the material types, achieved an average accuracy of 92.4%, while the proposed method improved the average accuracy to 98.7%.
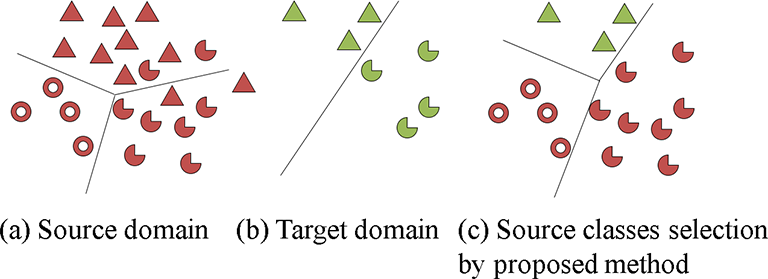
Before transfer learning, there are (a) source and (b) target domains. (c) By selecting useful source classes, discriminative representations can be learned.