Metallurgical Abstracts on Light Metals and Alloys vol.55
Achieving superconductivity with higher Tc in lightweight Al-Ti-Mg alloys: Prediction using machine learning and synthesis via high-pressure torsion process
Masaki Mito1, Narimichi Mokutani1, Hiroki Tsuji1, Yongpeng Tang1, Kaname Matsumoto1, Mitsuhiro Murayama2,3 and Zenji Horita1,4,5
1Graduate School of Engineering, Kyushu Institute of Technology, Kitakyushu 804-8550, Japan
2Department of Materials Science and Engineering, Virginia Polytechnic Institute and State University, Blacksburg, Virginia 24061, USA
3Institute for Materials Chemistry and Engineering, Kyushu University, Kasuga 816-8580, Japan
4Magnesium Research Center, Kumamoto University, Kumamoto 860-8555, Japan
5Synchrotron Light Application Center, Saga University, Saga 840-8502, Japan
[Published in J. Appl. Phys. Vol. 131 (2022), 105903]
https://doi.org/10.1063/5.0086694
E-mail: mitoh[at]mns.kyutech.ac.jp
Key Words: Al-Ti-Mg alloys, superconductivity, machine learning, high-pressure torsion
Aluminum (Al) and titanium (Ti) are superconducting materials but their superconducting transition temperatures (Tc) are quite low as 1.20 and 0.39 K, respectively, while magnesium (Mg) never exhibits superconductivity. In this study, we explored new superconductors with higher Tc in the Al-Mg-Ti ternary system, along with the prediction using machine learning. High-pressure torsion (HPT) is utilized to produce the superconducting states. While performing AC magnetization measurements, we found, for the first time, superconducting states with Tc = 4:0 and 7.3 K for a composition of Al:Ti = 1:2. The magnetic anomalies appeared more sharply when the sample was processed by HPT at 573 K than at room temperature, and the anomalies exhibited DC magnetic field dependence characteristic of superconductivity. Magnetic anomalies also appeared at ~55 and ~93 K, being supported by the prediction using the machine learning for the Al-Ti-O system, and this suggests that Al-Ti oxides play an important role in the advent of such anomalies but that the addition of Mg could be less effective.
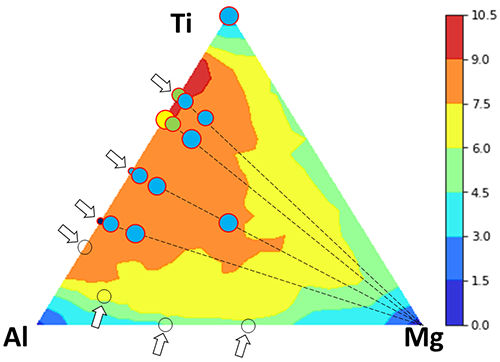
Mapping of Tc in an Al-Ti-Mg triangle, predicted by machine learning. The arrows indicate the compositions corresponding to the stable phases. The red circles represent samples prepared via the HPT processing.